Process system
simulation
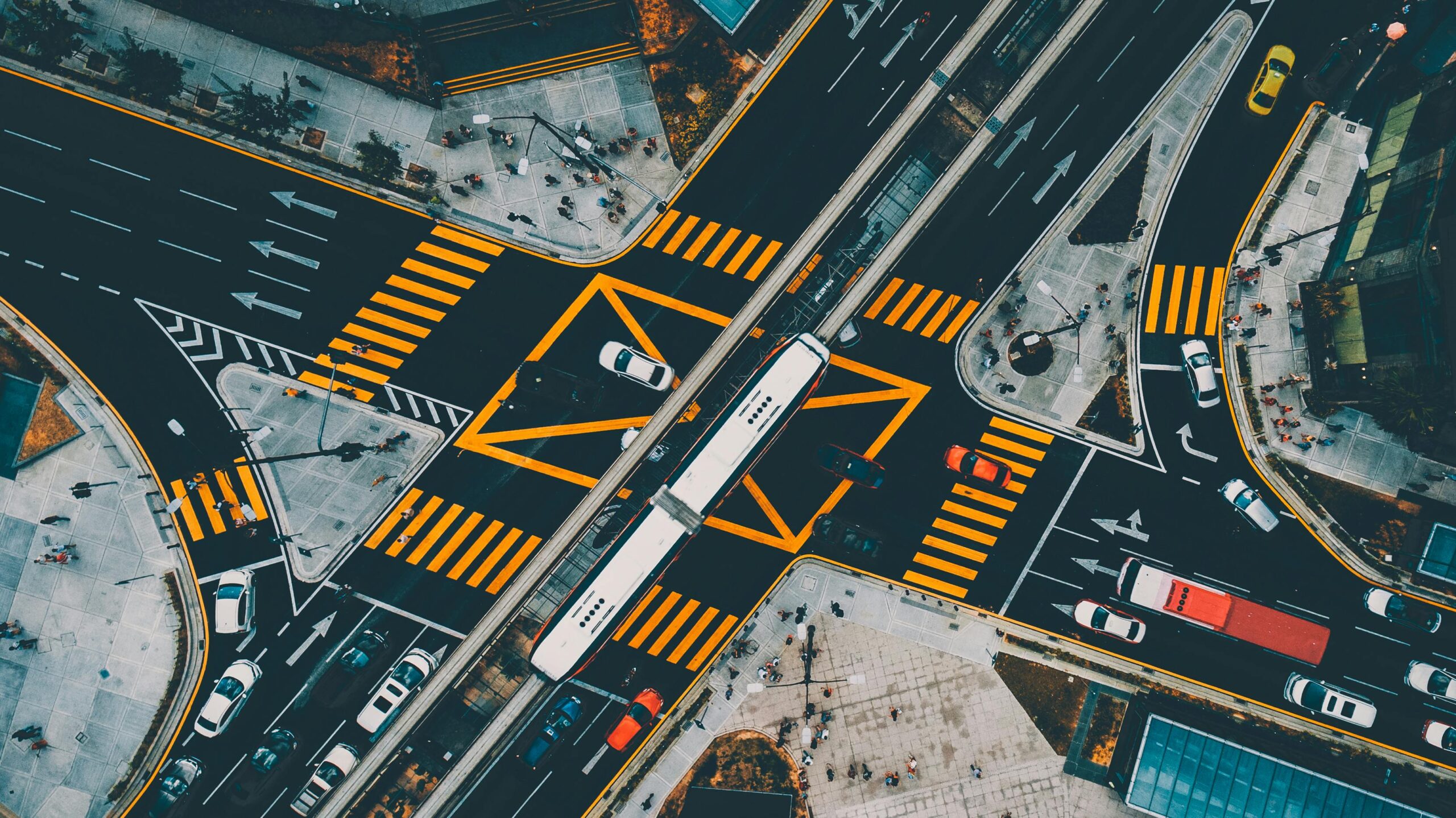
We develop simulation tools for industrial or service processes, introducing the inherent stochasticity to support decision making with statistical rigor.
Through systems based on discrete event simulation, it is possible to:
We use specific discrete event simulation tools in conjunction with ad-hoc developments to faithfully represent complex processes.
Benefits and potentials
- Simulate multiple scenarios and operating conditions to evaluate the impact of possible changes.
- Study changes in production systems before making investments, reducing errors and enhancing projects.
- Support decision making with strong evidence and statistical rigor.
- Represent variability in processing, waiting and set-up times to capture the stochastic nature of production systems.
- Generate reports and visualizations to better understand system behavior and communicate results to stakeholders.
Advanced data analytics
Processing large volumes of data from various sources (sensors, GPS, logs) enables rigorous statistical study of processes. This is a key input for simulation tools.
Our approach
Our approach focuses on modeling and analyzing the behavior of systems in different scenarios, enabling informed decision making and improving operational efficienc
1Stochastic processes
Most processes are stochastic in reality and involve variables or stages that are best described by means of random variables.
When you want to evaluate stock levels at critical points, fleet sizing, the effect of demand peaks, the dispersion in the times of any process, the flow of materials in production systems, the design of new production lines, among others, it is essential to address these problems from tools that take into account the inherent uncertainty in the processes, as well as exogenous variability.
When you want to evaluate stock levels at critical points, fleet sizing, the effect of demand peaks, the dispersion in the times of any process, the flow of materials in production systems, the design of new production lines, among others, it is essential to address these problems from tools that take into account the inherent uncertainty in the processes, as well as exogenous variability.
2Process modeling and simulation
We specialize in developing and modeling simulation processes based on rigorous methodologies that allow understanding the effect of process variability. We mainly use discrete event simulation and Monte Carlo simulation for these purposes, especially applied to understand complex processes.
3Scenario evaluation and optimization
These types of methodologies allow stakeholders to evaluate multiple What-If scenarios, varying structures, designs, resources, timing, among other variables to see the effect on key process indicators.
4Impact also on services
A service can also be modeled through these techniques, understanding the resources associated with each stage, the expected queues, seeking to maximize the level of service and minimize the resources affected. Working with large volumes of data is key to be able to statistically represent each sub-process in a rigorous way.